How Sustainable are AI Policies?
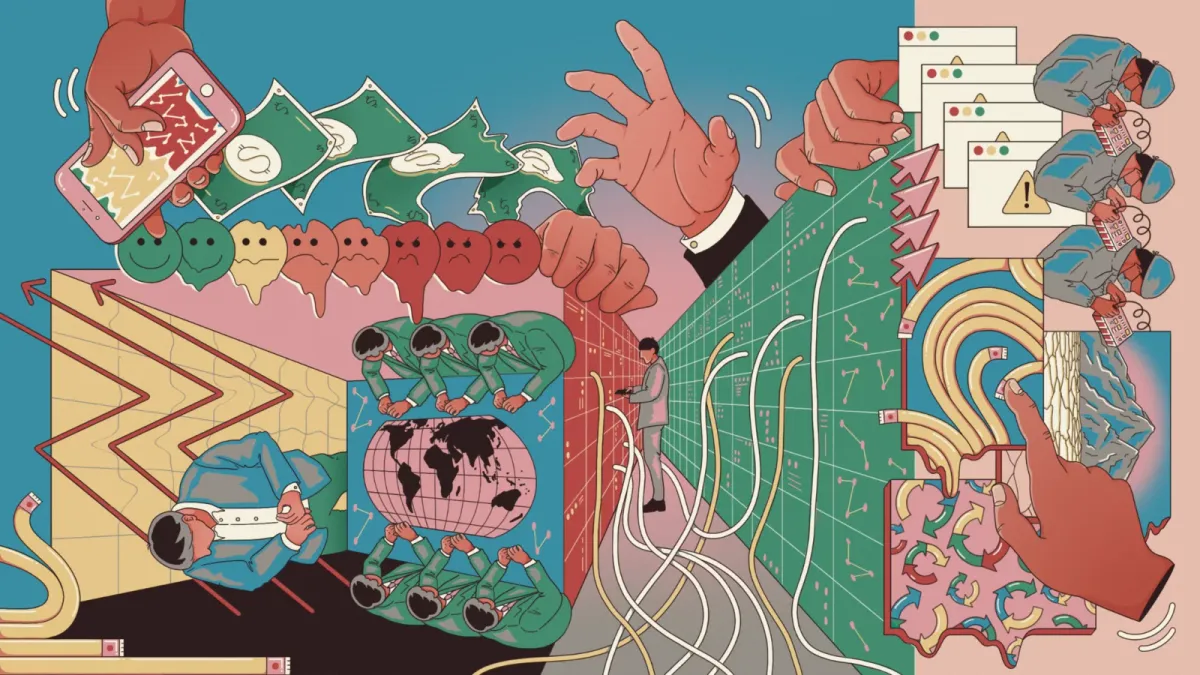
Setting the tone for a Sustainable AI Policy Series:
This inaugural article is part of a series on the theme of Sustainable Artificial Intelligence (AI) Policies that attempts to methodically answer this apparently simple question. As a researcher working on the domain of AI policy and governance, I felt a compelling need to unspool the various threads that make a messy knot called Sustainable AI. I am Sreekanth, and I will take you through the complex evolving terrain of AI policy making and try to go beyond the usual policy discourses on Sustainable AI. At the outset, I would like to acknowledge my time spent at CAIS as a fellow (2022-2023) where many of the initial ideas took root. In this background piece, I set the stage for the forthcoming articles that will constitute a series.
In this series, I will initially explore the Sustainable AI discourse and framework through three case studies viz., China, the EU, and the USA. These case studies are selected based on three major criteria: 1) the size of their economy, 2) the size of their digital economy, and, 3) having an AI strategy/policy/regulation published by a government agency. Over time the series may include other countries which are at different stages of AI policy and regulatory development.
Over the course of the next few months, I will publish a series of articles on how the AI national / regional strategies, policy frameworks and regulatory actions in these three select case studies deal with the issue of sustainability. While the prognosis will mainly focus on the environmental aspects of their AI policies, it will also go beyond the dominant framing of sustainable AI, casting a critical lens on the socioeconomic and political factors that inflect the making of these AI policies. As stated above, the project presents a multidimensional evaluation of how different AI policy frameworks deal with the sustainability question but it will do so by keeping the issues of rights, equity and justice at the core of the evaluation.
Making sense of 'Sustainable AI'
Artificial Intelligence is the buzzword that we hardly escape hearing. The advancements in Machine Learning (ML), availability of massive data and large scale investments in building compute infrastructure such as semiconductors, cloud and data centers have made AI the most propitious technology that can purportedly solve a wide variety of societal and market challenges. The promising future potential of AI in achieving higher productivity, efficiency gains and even replacing or augmenting human cognitive capacity is so tantalizing that both commercial and policy actors are pushing for further acceleration of its development and deployment. This has given rise to a race to gain AI supremacy, consequently making it a strategic imperative to achieve national competitiveness.
Responding to the rapid developments in the field of AI, including recent advancements resulting in proliferation of transformer based language models (Generative AI), there have been significant efforts to understand, regulate and mitigate potential risks, power imbalances and ethical implications that these technologies could pose. At the same time, a strong discursive framing has emerged around AI’s prospects in achieving UN Sustainable Development Goals (UN SDGs), promoting innovation-led growth, to advance citizens’ welfare and acheiving environmental sustainability. The acceleration of AI’s development and its deployment is seen as not just a business growth imperative but also a policy imperative that is necessary to achieve sustainable development goals.
This series will therefore examine the policy and market environment that precipitates AI acceleration, and assess the ensuing regulatory actions focusing on sustainability in major AI adopting geographies.
AI Efficiency Paradox
AI is increasingly framed not only as a technological concept but also as an ideological and a marketing concept. This positions AI as a general purpose technology promising efficient solutions to market and social challenges alike. However, the advancement of AI, particularly in deep learning, presents a critical paradox in environmental sustainability. While AI technologies are perceived to offer substantial efficiency gains across sectors, the resource-intensivity involved in every step of AI development lifecycle processes may ultimately undermine these benefits. This rebound effect dynamic eerily mirrors the Jevons Paradox, an economic principle named after William Stanley Jevons.
Jevons observed that technological advancements leading to increased resource efficiency (e.g., the steam engine for coal) could paradoxically lead to increased overall consumption due to factors like affordability and the creation of new applications. A recent research has exposed the environmental cost of AI development. Training a single large language model can generate around 600,000 lbs of carbon dioxide. Google's AlphaGo Zero, for instance, produced 96 tonnes of CO2 during 40 days of its research training, equivalent to the footprint of 1000 hours of air travel.
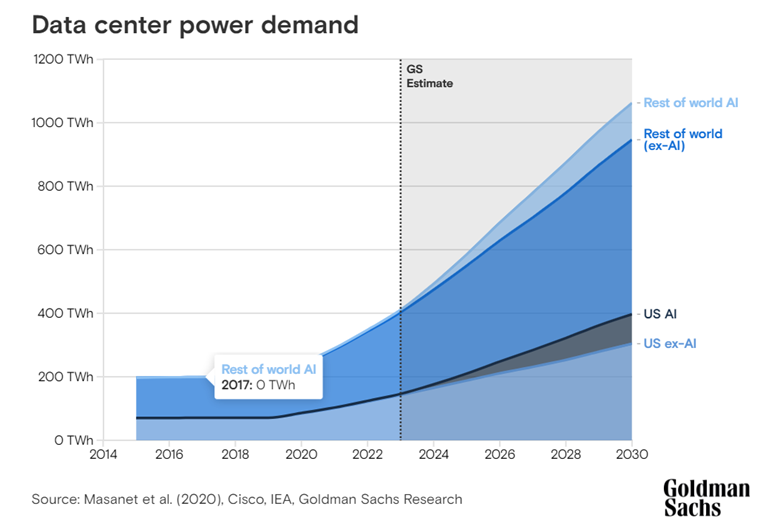
This high energy and water consumption stems from two key factors: First, training sophisticated AI models demands immense computational power, typically supplied by energy-intensive data centers. Second, the industry's pursuit of enhanced AI capabilities drives continuous advancement in semiconductor technology, creating a complex feedback loop. Manufacturing these increasingly advanced chips requires exponentially more energy and water resources. As AI development pushes the boundaries of chip performance, the environmental cost of semiconductor production rises, perpetuating the demand for even more powerful processors. This cycle is further constrained by fundamental physics - while chip miniaturization has traditionally improved efficiency, shrinking memory cells beyond certain nanometer thresholds actually reduces energy performance. Modern chips may offer unprecedented computational power, but the energy required to move data between their densely packed components often offsets these efficiency gains. In early 2024, news reports claimed that Sam Altman, CEO of OpenAI was seeking to raise a whopping US$ 7 trillion investments to realise his AGI (Artficial General Intelligence) dreams by building enormous chip building capacity.
New evidence suggests that beyond energy, the environmental impact of AI also extends to water usage. Kate Crawford asserts that Generative AI systems require vast quantities of fresh water for processor cooling. A lawsuit revealed that a data center supporting OpenAI's GPT-4 used a staggering 6% of a district's water in Iowa (just before training completion). She observed similar trends with Google and Microsoft's large language models (Bard and Bing), with environmental reports showing water use spikes of 20% and 34% respectively. These examples highlight the potential scale of the problem, with some estimates suggesting global AI water demand could reach half of that of the United Kingdom by 2027.
Current State of "Sustainable AI"
The prevailing narrative of "AI for Sustainability," emphasizes AI's potential in combating climate change, optimizing energy usage, and resource management. However, concerns arise regarding increased carbon emissions from AI's energy-intensive processes, such as training ML models and neural networks. Most assessments however, focus on the direct environmental impacts of AI compute, neglecting indirect consequences stemming from AI applications.
The current discourse within the sphere of Sustainable AI has two framings: 1) addressing the environmental impacts of AI; 2) utilizing AI to combat the climate crisis. Aimee van Wynsberghe's work, Sustainable AI: AI for Sustainability and Sustainability of AI sheds light on both these perspectives. In his 2022 paper, Peter Dauvergne critically questions the "AI for Ssustainability" frame and argues that deploying AI technologies have accelerated extraction across many sectors and turbocharging consumerism.
Additionally, studies such as The Role of Artificial Intelligence in Achieving the Sustainable Development Goals evaluate AI's role in advancing or hindering SDGs, particularly the environmental goals (SDG 13, 14, and 15). While some findings suggest AI's potential benefits outweigh inhibitory factors (as the graph below shows), caution is warranted due to potential biases in existing research.
The above research suggests that only 30% targets under the environment pillar are inhibited by AI. However, recent research argues that this assertion is based on previous literature research that may be biased to the positive reporting of AI impact and the analysis of environmental impact only count instances of impacts, but do not consider the scale or their possible indirect effects.
More recently, focus has increased towards evaluating AI's environmental impact and proposing policy measures to mitigate climate risks associated with AI. In the last couple of years there have been considerable efforts to measure the impact of AI development life cycles, right from mineral extraction to infrastructure build up, and compute to application. Serious attempts are underway to provide necessary knowledge to policy makers and commercial entities about the sustainability challenges prevalent across the AI development lifecycle.
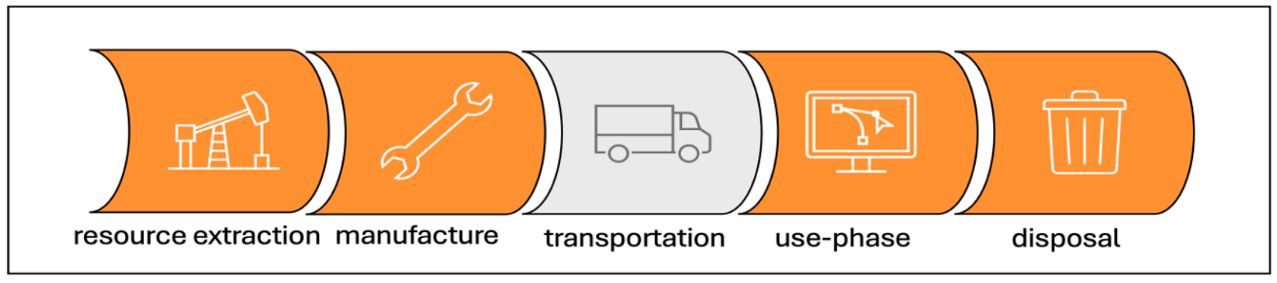
In late 2022, two significant papers were published to measure the imact of AI on environmental sustainability. One estimated the carbon footprint of BLOOM, a open source large language model. The other was published by OECD to help measure the impact of AI compute and applications on the environment. Notably, German-based research institutions, Institut für ökologische Wirtschaftsforschung and AlgorithmWatch’s SustAIn project published a joint report prioritizing sustainability as the fundamental value for assessing AI. Looking critically at the inevitability of AI development to address societal challenges, it drew attention to environmental consequences, including carbon emissions, electricity consumption, mineral mining, land, and water usage, and electronic waste generation. These impacts disproportionately affect marginalized communities, highlighting the policy lacuna in addressing the adverse challenges across the lifecycle of AI development. Another paper by researchers from University of Bonn explicates how every stage of AI development lifecycle pushes planetary boundaries there by pose serious challenges not just for wellbeing of people but the sustainability of the planet.
Geopolitical shifts in mineral extraction reflect a trend towards resource nationalism, driven by climate concerns and global supply chain disruptions. This dynamic reshapes mineral trade patterns, yet retains colonial legacies of exploitation and dependency. Understanding the coloniality of the AI supply chain requires examining its material infrastructure, environmental costs, and socio-political implications, including rigorous data collection and analysis involving local communities. The negative environmental effects of AI compute, mainly during production, transport, operations, and end-of-life stages, will be highlighted in this series.
Towards Sustainable AI Governance
It is encouraging to see that the environmental impact of AI is gaining increased traction among academics, civil society groups and policy makers. However, in the current AI policy discourse and governance, ethical AI frameworks like "Responsible AI" and "Trustworthy AI" are embraced by commercial enterprises, think tanks and governments, denuding their original meaning and often ignoring the negative environmental impact of AI systems. The global landscape for climate policy is becoming increasingly complex and uncertain. The return of the Trump administration in Washington, coupled with ongoing geopolitical conflicts and the intensifying technological rivalry between major powers, are overshadowing comprehensive climate transition initiatives. These competing priorities are diminishing the intent of integrating climate justice into the AI policy formulation.
This research series will provide a comparative analysis of AI governance frameworks, examining their sustainability across three major AI-adopting regions: China, the EU and the USA. Through systematic evaluation of current and proposed policy and regulatory measures, the series will assess how well these frameworks deal with environmental impact and related societal challenges. The evaluation will be carried out using four key parameters as stated in table 1 below.
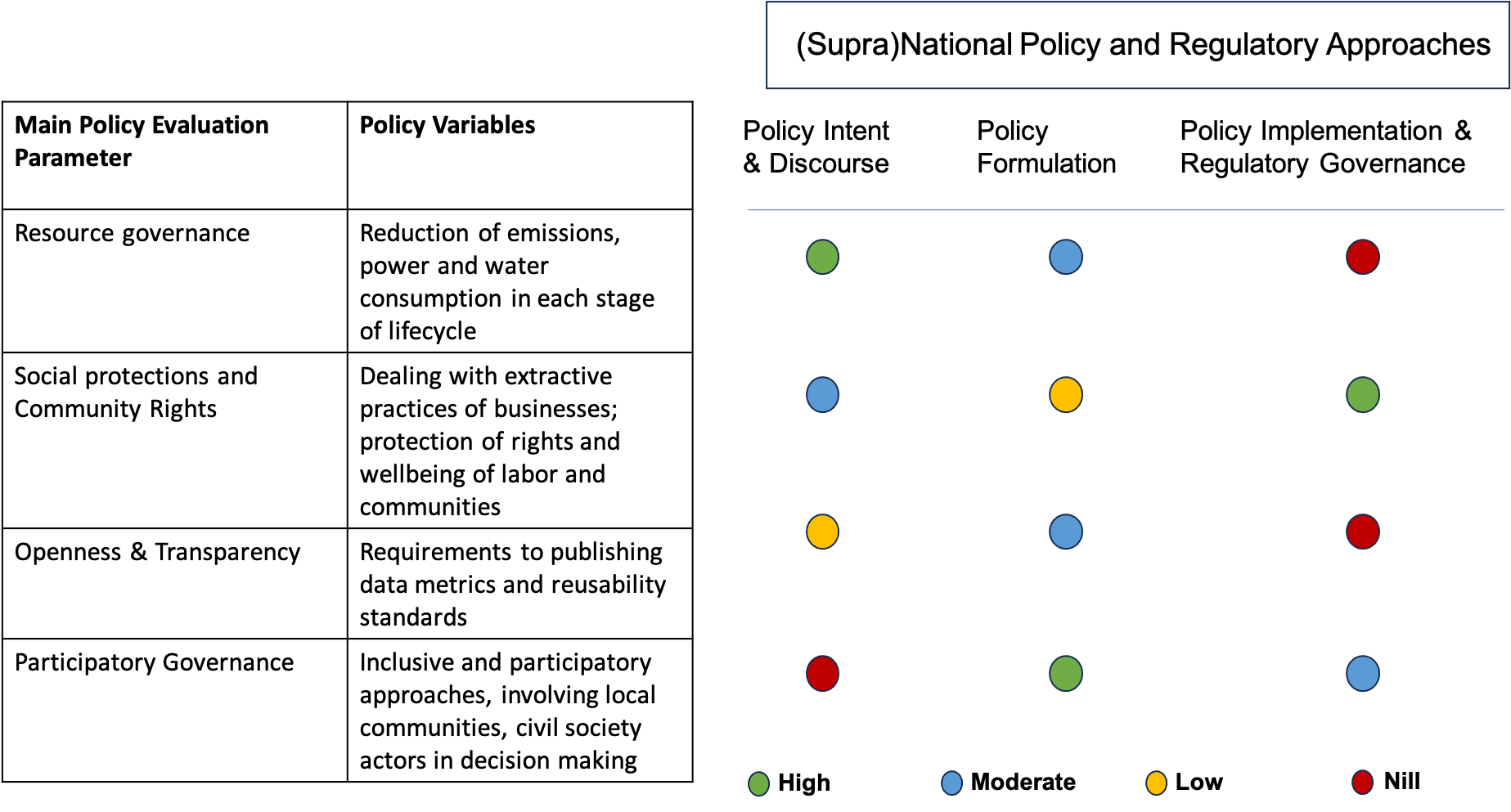
Based on the above illustrated evaluation parameters, the series aims to fill notable policy gaps in the prevalent sustainable AI policy/governance discourse and frameworks, by integrating the concerns surrounding AI governance into mainstream discussions on environmental sustainability. The article series will therefore critically evaluate the sustainability of AI policy and regulatory proposals as well as legislations shedding light on the need for a multidimensional approach to evaluate AI policies that encompass both ethical and environmental dimensions to ensure a just, equitable and sustainable AI future.
This article series is a result of my work during the fellowship at the Center for Advanced Internet Studies (CAIS Research).
